As forensic science adopts single nucleotide polymorphism (SNP) testing and expands its toolkit, certain techniques developed in other genomic fields—such as imputation—also are being considered to increase testing success. Imputation, a method for inferring unobserved genetic data, has been used in medical and population genetics studies to extend analysis capabilities. However, applying this technique to forensic genetic genealogy presents unique challenges and considerations. This post explores the origins and mechanics of imputation, how it can support forensic investigations, and the potential pitfalls when applied to sparse or low-quality forensic data.
Traditional Applications of Imputation
Imputation can be used to infer missing genetic information by leveraging patterns observed in large, comprehensive datasets. Initially adopted in human population studies and medical genetics, imputation has enabled researchers to extend datasets by “filling in” missing genetic markers based on data from representative reference panels—essentially large-scale databases representing diverse population genetics information. By doing so, scientists have been able to increase the resolution of studies without the need for additional genotyping.
At its core, imputation relies on genetic and statistical models that infer missing SNPs or other genetic markers based on patterns within observed data. If a certain SNP, in a reference panel, is present in individuals with similar genetic backgrounds in a reference panel, imputation software might infer that same SNP in an individual of similar genetic background who was not directly genotyped for that SNP or set of SNPs. The accuracy of these inferences largely depends on the density of observed data points and the quality and representativeness of the reference panel(s). In population studies where imputation has proven valuable, the studies use high-density data across individuals with shared ancestries, to produce estimates of missing genotypes.
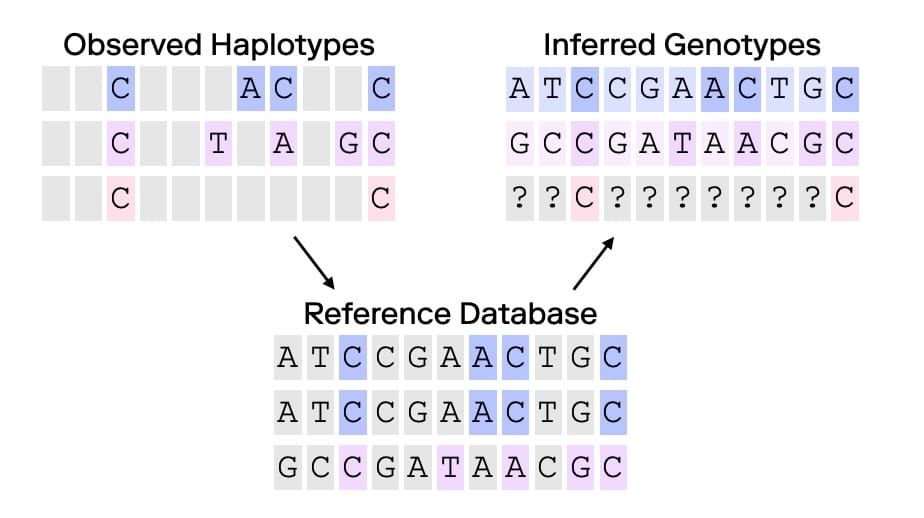
Can Imputation Benefit Forensic Genetic Genealogy?
In forensic genetic genealogy, imputation may yield genotypes when only partial or low-density SNP data are available, such as when analyzing degraded samples or low-coverage data. Imputation can enhance data completeness by filling in missing SNPs, making a partially degraded genetic sample more comparable to profiles in genetic genealogy databases. This process aligns forensic samples with consumer databases, many of which are built on SNP microarrays that capture common SNPs. While imputation can improve compatibility, it comes with substantial risks that must be considered before applying this tool. Indeed, newer forensic matching systems, such as Multi-Dimensional Forensic Intelligence (MDFI), are designed to handle incomplete SNP profiles effectively without relying on imputed data, thereby reducing the associated risks with imputation. Because imputation can introduce errors, especially when based on sparse data, it always is better to rely on directly observed information. Forensic scientists should select methods that generate dense SNP data, i.e., obtaining as much coverage and read depth as possible, as opposed to invoking imputation as a work around or "hack".
Limitations and Risks of Imputation in Forensic Applications
Imputation comes with limitations that are particularly relevant to forensic science:
-
Reliance on Dense, High-Quality Data: Imputation performs best when working with high-density SNP profiles, such as those obtained through high-coverage whole-genome sequencing. When data are too sparse—like low-coverage sequencing or highly degraded samples—the imputed information can lack accuracy, creating “imputed noise” rather than valuable data. In forensic cases, where a limited amount of evidence exists, inaccurate relationship predictions can result which in turn can lead to costly investigative misdirection(s).
-
Quality Control and Uncertain Reliability: Forensic genetic genealogy requires rigorous quality control due to the high-stakes nature of the results and investigative outcomes. Although imputation has a solid foundation in medical research, its application in forensics is newer, the risks are not well understood, and it may not yet have the reliability needed for or be defensible within legal contexts. Without sufficient accuracy and demonstrated reliability and validity, imputation could mistakenly inflate the apparent genetic closeness between individuals, leading to false leads.
-
Challenges with Rare Variants: Standard SNP arrays focus on common SNPs that are well-represented across populations. However, forensic cases often benefit from the inclusion of rare variants and other genetic features such as small insertions and deletions (indels) that contribute to generating highly individualizing information. Imputation typically cannot infer these rarer variations with high confidence, especially if these other marker classes are absent from the reference panels. This gap underscores the need to drive towards directly observed, comprehensive data in complex forensic investigations.
-
Challenges with Admixed or Under-represented Ancestries: Imputation accuracy can be especially compromised in cases involving admixed individuals, where a person’s genetic ancestry comes from multiple populations. Admixture introduces diverse haplotypes from different ancestral backgrounds, complicating inferences based on reference panels that may not adequately represent mixed genetic backgrounds. When populations with distinct haplotypes are combined in an individual’s genome, imputation algorithms may struggle to accurately infer missing SNPs, potentially leading to incorrect results. For forensic genetic genealogy, this limitation underscores the need for diverse, representative reference panels and for extreme caution when interpreting imputed data for individuals of mixed ancestry.
The Importance of Data Density: Observed vs. Imputed Data
One critical factor to support the success of imputation in forensic genomics is the density of directly observed SNP data. Dense, high-quality data provide a foundation for accurate imputation. In contrast, sparse data lack the contextual information needed to reliably infer missing genetic markers. Forensic samples, especially those from degraded or ancient remains, often have sparse data that may render imputation unreliable. When raw data are minimal, imputation becomes less of a supportive tool and more of a liability, potentially introducing inaccuracies that compromise genetic profiling and case outcomes. High-quality, directly observed SNP data always should be sought for forensic genetic genealogy, even as imputation technology advances.
Moving Forward: How to Integrate Imputation Wisely in Forensics
For imputation to be a reliable tool in forensic genetic genealogy, it should be applied with caution and used only in situations where data density supports its accuracy. Some considerations for its responsible use are:
-
Prioritize Data Quality: Where possible, obtain the highest quality and coverage of SNP data. This tenet ensures that imputed SNPs are based on a solid foundation of observed data.
-
Limit Use in Sparse Datasets: Avoid using imputation extensively in cases where the genetic data are too sparse. Instead, focus on gathering additional, high-quality observed data to improve reliability.
-
Regularly Update Reference Panels: The effectiveness of imputation hinges on the quality of reference panels. As genetic research continues to expand, regularly updating these panels with diverse, population-representative data can improve the accuracy of imputation efforts.
Balancing Observed and Imputed Data in Forensic Genetic Genealogy
Forensic scientists and investigators must be aware of the risks and limitations of genomic tools, especially when leveraging tools from adjacent disciplines for use in forensic DNA testing. In forensics, imputation should be used sparingly and applied only where data quality and density allow for reliable analysis. Nothing replaces the value of high-quality, directly observed data.
As forensic genomics continues to evolve and continues to demand the highest standard of accuracy and quality, balancing use of imputation with rigorous quality control that properly considers risk and uncertainty will be essential to maximize its benefits and avoid misinterpretation in investigations.
Our research team at Othram invests heavily in understanding and measuring the performance of various tools and methodologies in forensic DNA testing. The measure of performance in forensic genetic genealogy is ultra-sensitive DNA profiles capable of detecting distant genetic relationships. This process requires obtaining lots of correct data from forensic evidence and as little incorrect data as possible. Often the difference between a solved case and a DNA dead end is in the details; the more genetic details available, the better is the chance of developing a viable investigative lead.
If you are not ready to onboard this new technology in your own forensic setting yet, come to Othram. Our team operates the world's first purpose-built forensic laboratory for forensic genetic genealogy. We developed Forensic-Grade Genome Sequencing® or FGGS® to enable ultra-sensitive detection of distant relationships. It's part of our Multi Dimensional Forensic Intelligence (MDFI) platform.
More forensic genetic genealogy cases have been solved with Othram FGGS® than any other method. Let’s work together to unlock answers and bring justice to those who need it most. Get started here.